AI Video Enhancement: Implications on Forensic Validity
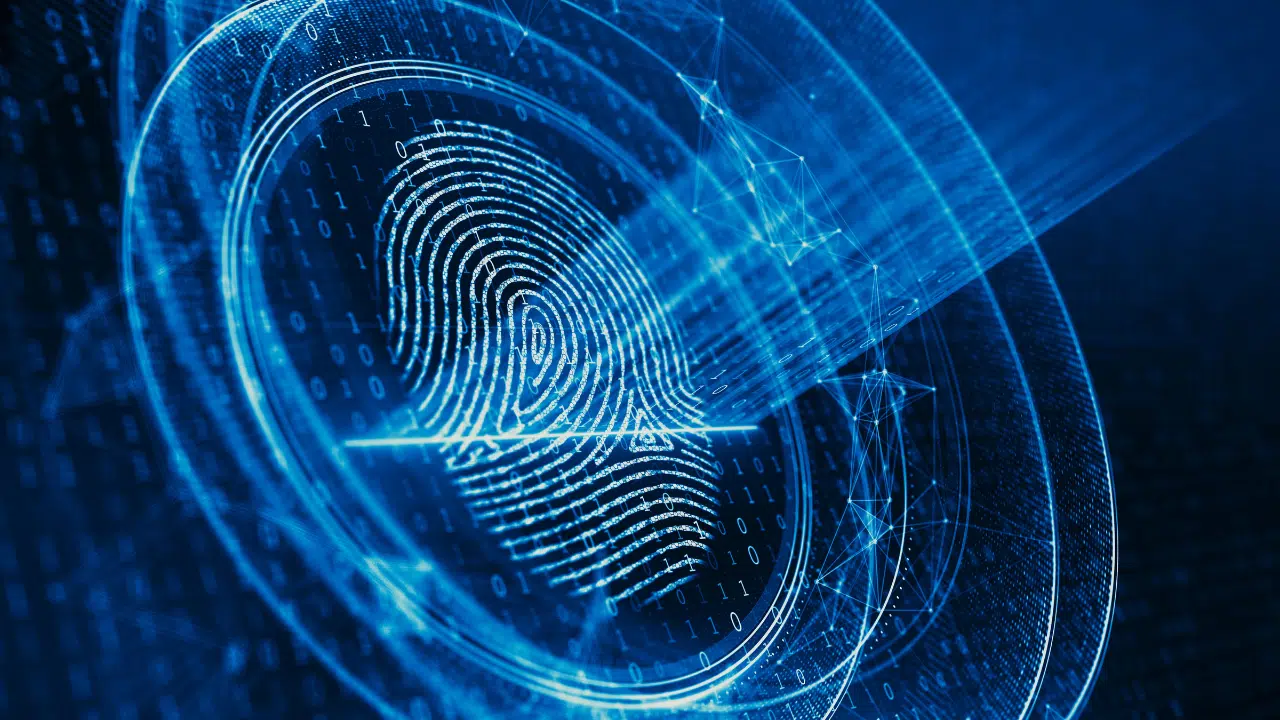
Artificial intelligence (AI) has revolutionized the way cameras process and enhance images, raising concerns about forensic authenticity. As AI-driven enhancements become commonplace in forensic science, surveillance, and security applications, a critical question arises: does introducing AI-powered algorithms to video footage compromise its evidentiary integrity? The answer lies in understanding the distinction between the broad range of image enhancement functions and the algorithmic techniques applied to the source —from de-noising and sharpening to re-coloring, inpainting, and up to full image (and video) generation.
AI and Forensically Authentic Images
Recent advancements in AI have integrated intelligent image processing into the vision pipeline, improving video quality by reducing noise, enhancing sharpness, and refining details. However, this has triggered debate about whether such enhancements alter the original scene in a way that affects forensic validity.
For an image or video to be considered forensically authentic, it must:
- Accurately represent the original scene without material alteration.
- Maintain a verifiable chain of custody from capture to presentation.
- Preserve intact metadata to indicate any modifications.
- Undergo technical validation to confirm that no unauthorized alterations impact its evidentiary significance.
In court settings and forensic investigations, authenticity often relies on establishing:
- The photo’s provenance (who took it, when, where, and with what equipment)
- That it has not been altered in ways that change its evidentiary significance
- That proper handling procedures were followed to maintain integrity
Organizations like the Scientific Working Group on Digital Evidence (SWGDE) and National Institute of Standards and Technology (NIST) set guidelines for handling digital photographic evidence, while industry-wide initiatives such as C2PA.org work toward ensuring content authenticity. Hailo has recently joined C2PA to contribute to device-level authenticity attestation, to address points #3 and #4 above regarding metadata preservation and providing the means for technical validation.
The Difference Between AI Restoration and Generation In Forensics
At the core of AI-enhanced image processing is the goal of maximizing the signal-to-noise ratio (SNR) of the bitstream as obtained from the sensor. Simply put, there are two ways to achieve this: either by reducing the noise with minimal impact to the signal, an approach that is focused on image restoration, mainly through noise modelling. Alternatively, SNR improvement can be obtained by increasing the signal by enhancing the semantic content, a technique focused on image regeneration. This approach focuses on information extraction from the source, and as a result may also result in generating new content.
The distinction between these two approaches is crucial in determining whether AI-enhanced footage remains valid for forensic use.
In fact, this is not only a matter of determining whether AI related algorithms were applied to the final image, since classic processing pipeline also manipulates source samples in a way that generates a ‘modified’ version of the original signal. This is the case with e.g. 3DNR filter that is applied in order to unblur the image but may introduce unnecessary edges if not properly applied. Another example is gray-world assumption in AWB (Auto White Balance) that is applied in order to correct color inconsistencies but may result in unnatural coloring.
Restoration-Focused AI methods work within a defined mathematical model to remove noise, sharpen, or deblur an image without adding new content thereby restoring the original source signal. This allows an explicit mathematical representation of the original image in the problem statement. Regenerative AI, on the other hand, uses a scheme in which the output is recovered from zero-knowledge source with some guidance (conditioning) signalled from the origin, filling in gaps with newly created elements. These approaches tend to re-create a different version of the original input through distillation of some properties, preserving some while removing others. Techniques such as variational autoencoders (VAEs) and stable diffusion models employ some encoding capacity for preservation of the desirable aspects of the inputs, while disposing some of the information, and then employ some decoding capacity to generate the final output, introducing new content that is non-existent in the original source signal. This poses a risk to forensic authenticity because the AI is not merely enhancing; it is adding / complementing details that were never captured or eliminating details that were captured.
Ensuring Forensic Integrity Through AI Hardware
The challenge in forensic validity is ensuring that AI-driven enhancements do not alter the evidentiary significance of footage. The role of hardware providers is critical in this regard. By clearly indicating whether restoration-focused or generative-focused AI techniques are used in each configuration, companies can guarantee that image integrity is preserved while enhancing quality for better usability. User may choose between different hardware configurations, and be assured that this selection will be clearly indicated in the output data. As long as no regeneration techniques are being used, it is likely that the neural network cannot introduce new content that is meaningful in a psychovisual sense.
To further ensure forensic validity, hardware solutions can incorporate secure attestations that prove only permissible AI-based restoration techniques were applied, while blocking any unauthorized content generation. These attestations serve as a cryptographic proof of an image’s provenance, acting as a digital stamp that verifies any generative operations occurred post-capture and were not approved by the hardware provider. This ensures that from the moment of capture to its final use, the footage remains unaltered in ways that would compromise its evidentiary significance.
Summary
As AI continues to shape the future of surveillance and security, maintaining the forensic validity of camera footage is essential. By differentiating between enhancement and regeneration, and by leveraging hardware-based authenticity seals, we can confidently integrate AI into security video systems without compromising the evidentiary trust in captured footage. In short, AI-enhanced does not mean AI-fabricated—and that distinction makes all the difference in forensic science.
At Hailo we are committed to put the control in the hands of our customers that build end products around the Hailo AI vision processors and will always guarantee transparent indication in product documentation when regenerative algorithms are engaged.
References
- Denoising: A Powerful Building-Block for Imaging, Inverse Problems, and Machine Learning (link)
- HDR image reconstruction from a single exposure using deep CNNs (link)
- Image-to-Image Translation with Conditional Adversarial Networks (link)
- High-Resolution Image Synthesis with Latent Diffusion Models (link)
- Pixel-Aware Stable Diffusion for Realistic Image Super-Resolution and Personalized Stylization (link)